- Ruhr-Universität Bochum
softTSense: Intelligent wastewater treatment with soft-sensor based real-time monitoring of the solids content.
In the sofTSense project we develop a soft-sensor for determining the composition of wastewater. A key characteristic of wastewater is its dry substance content, which has a decisive influence on the operation of wastewater treatment processes. The current state of the art in determining the dry matter content in wastewater is the analysis of samples in the laboratory, which leads to a significant time delay between sampling and the result, or the corresponding adjustment of the operation. This delay makes it difficult to effectively adjust the treatment processes to varying wastewater properties. Real-time detection of the dry matter content is an important key to the optimized operation of wastewater treatment in order to ultimately achieve cost, energy, and resource savings.
Specialized sensors for direct measurement of the dry matter content are a niche product and therefore expensive, and have also proven to be unreliable in on-site use. The use of soft-sensors primarily promises a reduction in costs due to less complex hardware. The central research question is whether soft sensors can also achieve sufficient accuracy in determining the dry matter content.
In general, soft-sensor technology is based on special algorithms that use a mathematical model of the physical process and a variable that is easy to measure to determine the actual process variable of interest in real time. To do this, the easy to measure quantity must have a physical coupling to the process variable of interest, and this coupling is described by the model. In the sofTSense project, the process variable of interest is the dry matter content, while the model structure and the quantities to be measured have not yet been ultimately defined, as this definition is one of the project’s research questions. Promising variables to be easily acquired during real-life operation are the easily accessible operating data of the pumps in wastewater treatment, which, for example, provide pressure measurements at various points in the process or record the motor electrical power virtually free of charge.
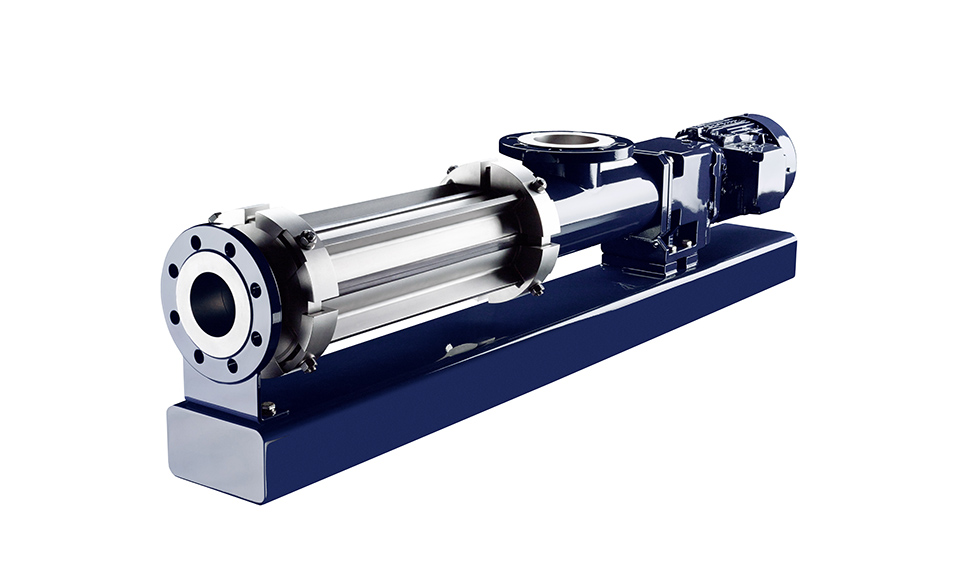
Figure 1: Progressing cavity pump manufactured by the project partner SEEPEX.
The project focuses specifically on progressive cavity pumps, as these are used at the relevant points in wastewater treatment. Progressing cavity pumps belong to the class of positive displacement pumps and are based on the principle of encapsulating a certain amount of fluid and transporting it mechanically from the suction side to the discharge side of the pump (see Figure 2). Highly viscous and multiphase fluids in particular, such as those occurring in wastewater treatment with occasionally very high dry matter content, can be transported efficiently in this way.
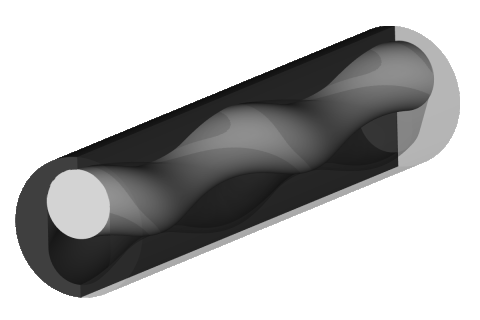
Figure 2: The operating principle of a progressive cavity pump is based on a specially shaped stator-rotor pair that forms chambers when the rotor rotates, in which the fluid is transported from the suction side to the discharge side.
The project initially focuses on model synthesis. A hybrid model structure consisting of first-order physical principles and data-driven approaches will be selected in order to make optimum use of the advantages of both modeling approaches. Validated models from a previous project are available, in particular for the mechanics of the progressive cavity pump. Machine learning methods are to be used to couple the mechanical models with the fluid properties and the flow conditions in the pump, which will be parameterized using the real-world and laboratory data provided by the project partners.
The soft-sensor resulting from the algorithms and models is to run on an embedded system directly on the pump in order to provide real-time determination of the dry matter content. The computationally intensive parameterization and the consideration of wear that occurs over time are outsourced to a cloud computing network.
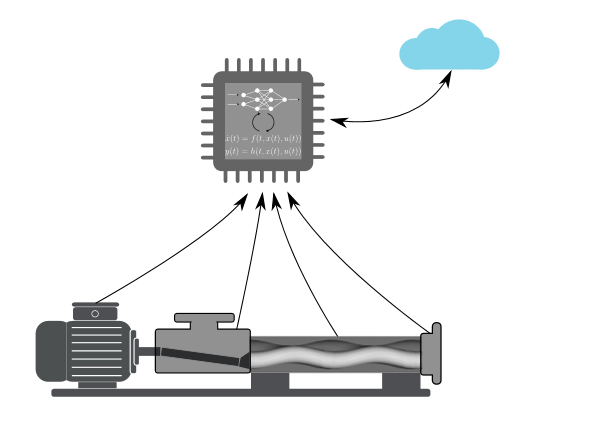
Figure 3: Sketch of a progressive cavity pump and the microcontroller on which the dry matter estimation algorithms are executed. Parameterization and prediction of long-term effects are outsourced in a cloud application.
The collaboration with the partners SEEPEX and Lippeverband builds on the successful cooperation within previous projects and continues the developments begun there.
Further information is available on the project’s website.